What are Antibody Libraries?
Antibody libraries are collections of unique antibody clones derived from B-lymphocytes of naïve or immunized donors. Upon exposure to antigens, antibodies undergo in-vivo or in-vitro affinity maturation and variable gene (V-gene) hypermutation to produce specific clones.1
These clones are inserted into a vector and expressed in their genotype through molecular techniques for therapeutic purposes.2 Display methods are used to expose antibody libraries to specific immobilized antigens followed by multiple cycles of elution and subsequent binding of antibody clones. This allows obtaining higher levels of specificity against target antigens.2, 3
Different sources and specific purposes dictate the basis of the construction of antibody libraries. They include immune, naïve, semi-synthetic and synthetic libraries. Immune and naïve libraries are generated only from naturally occurring sequences, whereas synthetic and semi-synthetic libraries are generated using computational and chemical methods.2
Key Considerations in Antibody Library Design
Key factors for designing mAbs and constructing antibody libraries include biophysical and chemical properties such as solubility, viscosity, glycosylation, amino-acid modifications, stability, and binding specificity when formulated against specific molecular targets. 4 Apart from decoding the genetic basis of antigen-antibody interactions, knowledge of molecular pathways, and the exact time and space for initial target interaction (extracellular space vs. cell membrane) are also critical in developing efficient mAbs. 5 Moreover, while developing antibody libraries, ensuring diversity also drives its success in clinical applications. 6
The main bottlenecks while designing diverse antibody libraries come from their sources, especially when natural sources are used. 8 A closer look at all these factors will elucidate the complexities involved in antibody library design and understand its underlying opportunities. Antibodies selected from an efficiently designed library with stringent biopanning conditions have higher affinities for their targets and are highly efficient. 3
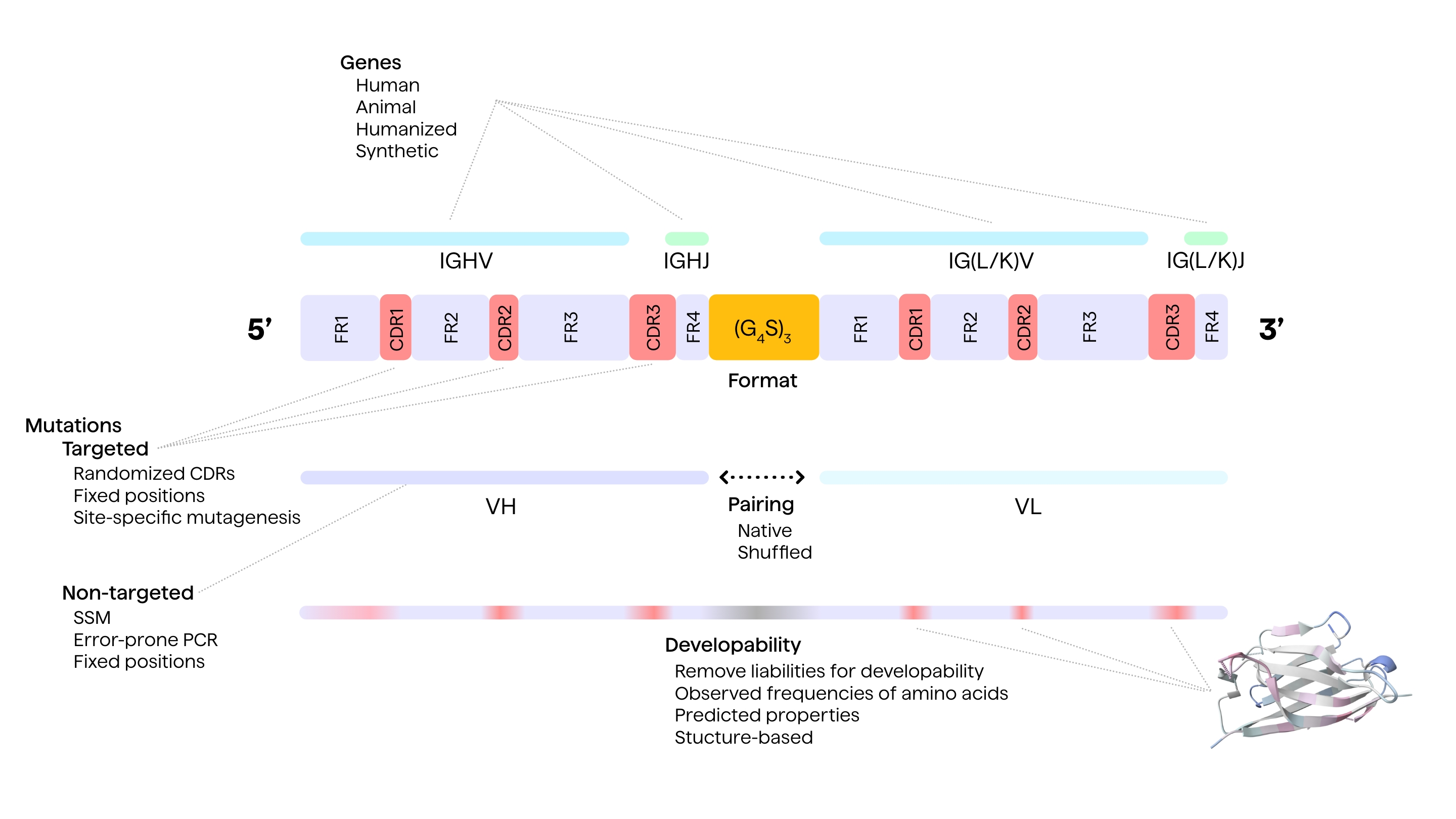
Design Approaches for Antibody Libraries
Choosing the target (antigen) product profile (TPP) and the generation strategy are two main approaches behind the successful design of therapeutic antibody libraries. A rational approach to antibody library design involves prior knowledge of the antibody structure (from either X-ray crystallography, nuclear magnetic resonance, or cryo-electron microscopy data), antibody sequence, antibody-antigen interactions, and their epitope-binding probabilities.7
Comprehensive knowledge about the complementarity determining regions (CDRs/paratopes), an idea of somatic hypermutations, the interactions between the Fab fragment and the epitopes, different amino-acid sequences, and their correlations to their locations in the antibody genes is also required. Minute structural details help in refining the efficacy and developability profile of the antibodies.8, 9
Types and Sources of Antibody Libraries
Naïve libraries are constructed from B-cell populations of non-immunized donors. In naïve libraries, antibody fragments (specific areas on the heavy and light chains) from donor B-cells are amplified using PCR in a series of reactions following which they are stored in plasmid vectors with their diversity assessed using NGS and validated using control antigens to assess their functionality against specific targets.10
Immune libraries are synthesized after immunization or exposure to an antigen, with knowledge of the specific target antigen. For example, the keyhole limpet hemocyanin (KLH) coupled p-nitrophenyl phosphonamidate (NPN) antigen for murine antibodies and specific viruses (e.g., Rabies) and tumor-specific sera for human antibodies.10
Once target-specific antibodies are synthesized using in-vivo and in-vitro methods, they can be mutated to create a new set of antibodies.
These antibodies can be genetically re-engineered and screened for potential binding properties against novel target antigens. This cycle is repeated multiple times to get a diverse and efficient antibody library. 8 Prevalent methods for construction of antibody libraries are combinatorial in nature and apply molecular engineering of antibody fragments from both human and animal sources where antibody genes are inserted into vectors. Antibodies can then be expressed and selected through display methods.
Major display methods include in-vitro phage display, ribosomal display, yeast display, and mammalian cell-surface display. 7, 11 Displayed antibody formats include single-chain variable fragments (scFv) or fragment antigen binding (Fab) regions from, for example. murine, camelid, rabbit or human hosts on the surface of bacteriophages, ribosomes, yeast cells or mammalian cell lines like HEK293 or CHO.
Designing Diverse in-vitro Antibody Libraries
The most common way to introduce diversity in an antibody library is to have a larger pool of unique sequences by carefully randomising amino-acid sequences of either an existing repertoire of pre-engineered proteins or from immunized donors.9 Diversity also depends on the number of donors, the type of donor tissue, the types of variable regions of an antibody from which these amino-acid sequences are amplified and the choice of V-gene frameworks used.17 Different display methods can then be used to express these diverse antibody libraries of different sizes.
Bacterial systems like E. coli can typically yield 109 colony forming units (CFU), and up to 1012 scFv of IgG using a thousand transformations, whereas ribosome concentration in cell-free systems can yield up to 1015 ribosomes.9
One can enhance the functional diversity of the antibody library by limiting the sequence randomization to only specific parts of the CDR region, introducing degenerate nucleotides along these selected CDR regions, using pre-defined CDR sequences, or even recombining naïve heavy and light chains.9
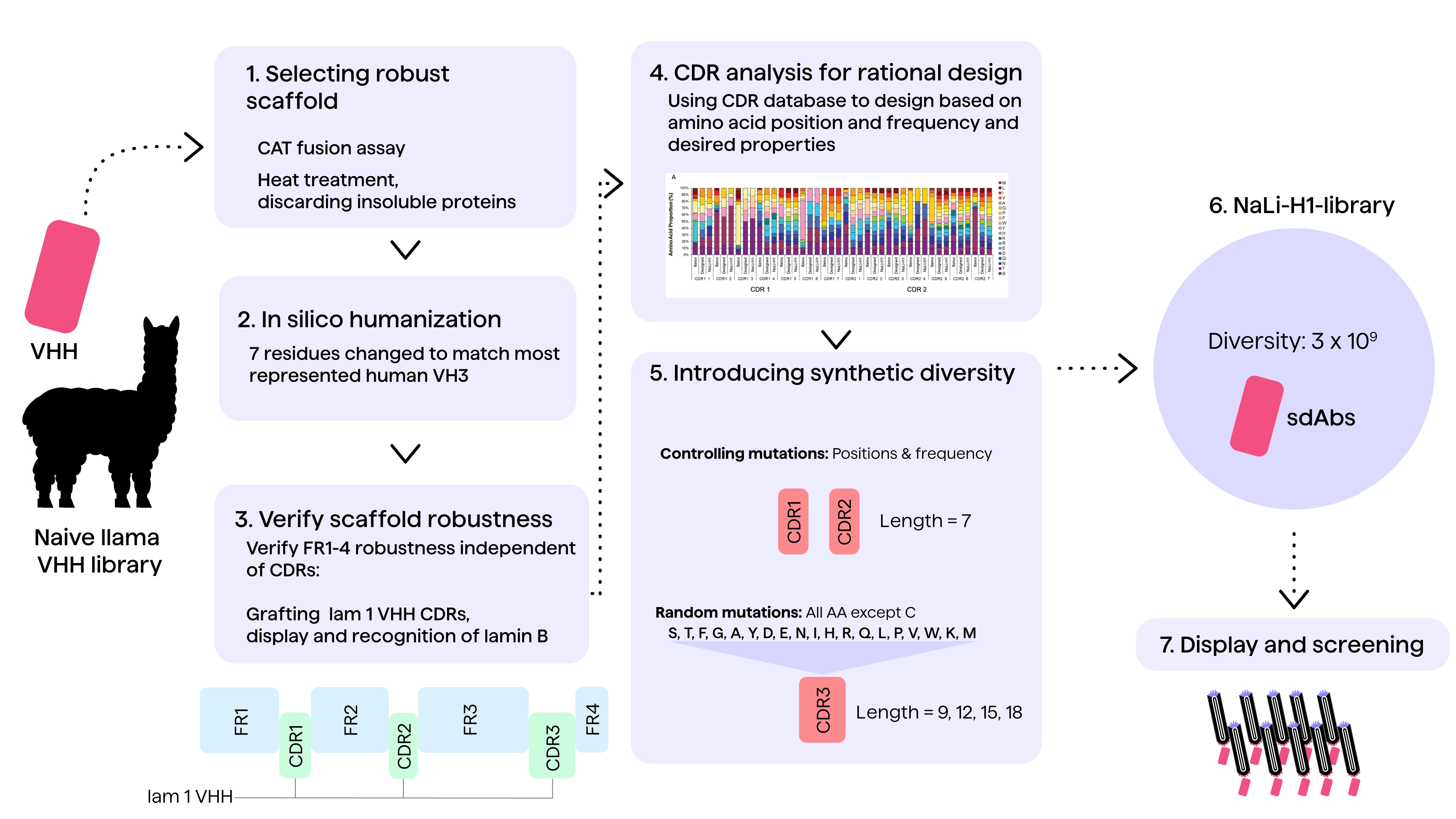
Non-targeted methods
Non-targeted methods for introducing mutations in an antibody library include error-prone PCR, chain shuffling, use of mutator E-coli, DNA shuffling by random fragmentation and site-saturation mutagenesis.10 Error-prone PCR can be used to induce mutations across the entire antibody gene thus producing a ready mutagenized library.18, 19
Error-prone PCRs are used in combination with ribosomal display methods since a PCR-based amplification is the first step of construction of ribosomal libraries and that it can generate clones of strengths 1012~15 without needing the transformation step.10
Using mutator bacterial strains like E-coli with phage display libraries can also be a viable method for inducing antibody diversity but it also mutates the vector backbone and thus needs subsequent re-cloning of just the antibody gene fragment.18, 20
Another method to induce diversity is to first fragment existing DNA pools and then introduce PCR (can be error-prone PCR or mutator bacterial strains) to amplify these fragments building a diverse library.10
Chain shuffling refers to the sequential shuffling of sequences in one of the heavy and light chain variable regions owing to their compatibility with multiple antigens and their poly-functionality. The shuffling is done with repertoires of V genes from unimmunized donors.21, 22
The main limitation with random mutagenesis is that it becomes difficult to locate the exact mutation responsible for binding affinity which makes deciding on the next steps for further mutagenesis to further enhance binding affinity, challenging.
Targeted methods
Targeted methods for introducing mutations include site-specific CDR mutagenesis and CDR walking applied to shorter, known target sequences. As suggested by the name, site-specific or site-directed mutagenesis refers to inducing mutations in known, target regions. Since the CDR regions are known to have the highest binding affinity, these regions have been traditionally targeted in site-directed mutagenesis.
However, some have preferred to preserve the VH CDR3/ LH CDR3 regions intact due to risks of losing binding affinity, and have instead targeted other CDR regions that might be useful in removing or reducing ‘low contact / repulsive residues’ with better kinetics and binding affinities.24 Site-directed CDR-specific mutagenesis is also less likely to induce immunogenicity in comparison to mutations in the more conserved regions.24
Site-saturation mutagenesis refers to the substitution of a single amino acid in any of the other 19 substituents which then leads to the formation of a library with a different set of mutated codons in the target positions.23 When done with alanine, it is also known as alanine scanning mutagenesis.
CDR walking helps in optimising the antibody binding sites by sequentially mutating the CDRs in a stepwise manner. After every round of mutation, the best mutant is used as the template for the subsequent round of mutagenesis and selection. This creates a more diverse and high-affinity batch of antibodies for libraries.25 Yang et al. developed a high affinity anti-HIV gp 120 Fab by the CDR walking strategy with a 420-fold increase in affinity (Kd=1.5x10-11 M) whereas Schier et. al. isolated an anti-c-erbB-2 scFv with picomolar affinity (Kd=1.3x10-11M) using CDR walking methods.25
Despite being more targeted and complimentary to immunization methods, the experimental setups and multiple iteration assays for in-vitro assays are tedious and require considerable amounts of resources for a marginal increase in antibody library diversity, and limited transformation efficiencies, especially in eukaryotic display vectors.10, 11
Another drawback of in-vitro designs of antibody libraries is the absence of somatic hypermutations (SHM) which is the key to adaptive, antibody-mediated immunity in host systems.10
Computational Methods for Antibody Library Design
The most modern methods applied in antibody library designs comprise in-silico computational techniques and more recently, implementing an ab initio approach, both of which are time and resource-efficient ways to get past the initial hurdles of antibody library design. Semi-synthetic and synthetic libraries are generated using computational methods.
Owing to decades of research on antibody generation, there are multiple data sources for information on antibodies in different domains in the present day and bioinformatic engineering techniques like homology modelling, protein–protein docking and interface prediction are useful in developing therapeutic antibodies.7 The most important of these are the Structural Antibody Database or the SAbDab, the Database for ImmunoGlobulins with Integrated Tools or the DIGIT, the Immune Epitope Database or the IEDB, and the International IMmunogGeneTics Information System or the IMGT.26
These databases provide crucial information to select the heavy chain and light chain variable region, optimise their orientation, selection of the CDR-H3 and non-CDR-H3 loops, and their optimization as well.8 Antibody numbering schemes like Kabat (number-based), Chothia (structure-based), and Aho (structure-based) help in annotating and reflecting structurally equivalent residue positions within an antibody sequence when performing sequence analysis.8
Four methods, namely OptCDR, OptMAVEn, AbDesign, and RosettaAntibodyDesign are used predominantly for ab initio design of antibodies based on antigen-antibody interface prediction. Antigen-antibody interfaces (CDR-paratopes) are determined using statistical approaches like Antibody i-Patch, Paratome or machine learning algorithms like proABC, Parapred, and Antibody Interface Prediction. Antibody-specific epitopes are identified using programs like ASEP, BEPAR, ABEpar, EpiPred, PEASE, and MabTope among others.7
Similarly, programs like ClusPro, SurFit, FRODOCK, and SnugDock are used for antibody-specific docking.7 ‘Hot-spot grafting’, a process wherein binding site motifs from existing protein–protein complexes are transferred directly onto an antibody and ‘re-epitoping’, where existing antibodies are tested for binding capacities towards target epitopes, are novel approaches to select best candidates (binders) for constructing the semi-synthetic and synthetic libraries, albeit needing further optimization.
Implementing machine learning (ML) algorithms and conducting computational mutagenesis of CDR3 regions have been used for optimization of designed antibodies for further iterations in in-silico modelling for antibody library synthesis. Such methods improve antibody stability and affinity through a combination of conformational and free energy change optimization upon modification of specific residues using programs like OptCDR, OptMAVEn, AbDesign, and RosettaAntibodyDesign.7
In order to identify potential binders and evaluate antibodies from experiments incorporating antibody libraries, PipeBio’s bioinformatic analysis platform can be used to effectively analyze B-Cell and T-Cell receptors (BCR/TCR), VHH, scFv and peptide sequences. There are comprehensive tools to automate workflows, identify sequences from large repertoires, identify potential liabilities and screen antibodies to screen therapeutic antibody libraries and identify antibodies with optimal binding affinity. All in one secure, centralized cloud platform.
Developability & Optimization in Computational Antibody Library Design
The ultimate challenge in antibody discovery and development is to identify developability factors in order to optimize mAbs for desired binding affinity, high specificity, excellent stability, and other favourable physicochemical properties for therapeutic applications, given that these traits are often conflicting from an evolutionary perspective, and inducing mutations to improve one of them often tends to worsen the others.11, 27
Excluding antibody sequences with liabilities like unpaired cysteines, deamidation hotspots or motifs related to non-specific binding, high viscosity and low solubility, is one way to improve developability of high-quality therapeutic antibody libraries.27 For instance, Teixeira et.al. embedded only pre-existing CDRs from natural antibodies into a genetically diverse panel of developable clinical antibody scaffolds to reduce liabilities.6, 27
Since the heavy chain CDR3 (CDR-H3) is known to be the most important in terms of binding affinity and specificity, these CDR-H3s were generated by PCR from B-cells from healthy donors, embedded with paired frameworks from previously validated therapeutic antibodies.6, 28
Another example is from a paper published in 2016 by Moutel et al.29, where the design and creation of one of the first synthetic libraries of humanized nanobodies is described (see Figure 2).
The library was constructed by using a strategy that included selecting for robust folding, controlled variability of CDR regions and low aggregation. First, a VHH scaffold displaying desired robustness was identified through screening of a naïve llama VHH library.
Subsequently, the scaffold was humanized by reducing the sequence distance between the camelid sequence and frequently observed motifs in human sequences. CDR grafting experiments were performed to validate the synthetic scaffold.
Computational methods with statistical scoring can be used to induce humanization and reduce immunogenicity in the resulting antibody sequences. The Humanness Score and Human String Content (HSC) are such methods. They are based on the sequence similarity between short, overlapping peptide sequences in animal-derived antibodies and the closest human antibody germline sequences.7 However, such scoring systems would need to be combined with structure-based design methods like re-surfacing (identifying solvent-exposed positions) and (ML) algorithms to predict T-cell epitope binding to MHC II complexes, to mitigate immunogenicity risks.7
Further optimization and risk mitigation procedures involve the identification of potential immune epitopes and aggregation prone regions (APRs) around the CDRs of the designed therapeutic antibodies. This approach also solves issues with the biophysical properties of the antibody like colloidal stability, solubility, viscosity, and pharmacokinetics.
Conclusion
Generating diverse antibody libraries with high binding affinities takes a combination of computational and in-vitro methods through multiple cycles of screening and optimization. Generation of monoclonal antibodies has come a long way from hybridoma technology, to display methods and now in their third generation, through computational assays.
On one hand, in silico methods make the initial design and ideation process time and resource efficient with known sequences and scaffolds for designing antibodies whereas advanced molecular techniques like cross interaction chromatography (CIC), hydrophobic interaction chromatography (HIC), stand-up monolayer adsorption chromatography (SMAC), affinity-capture self-interaction nanoparticle spectroscopy (AC-SINS), aid in assessment of the developability and optimization of generated antibodies.
With the immense amounts of data accrued from NGS of antibodies and emerging techniques like data mining, machine learning and high-throughput screening, antibody libraries can be designed, screened, and adequately interrogated paving a faster and more efficient path to generate novel, effective, therapeutic antibodies.